-
Notifications
You must be signed in to change notification settings - Fork 2
Commit
This commit does not belong to any branch on this repository, and may belong to a fork outside of the repository.
1. Doc updates. 2 Add a BO vignette.
- Loading branch information
Showing
11 changed files
with
285 additions
and
36 deletions.
There are no files selected for viewing
This file contains bidirectional Unicode text that may be interpreted or compiled differently than what appears below. To review, open the file in an editor that reveals hidden Unicode characters.
Learn more about bidirectional Unicode characters
This file contains bidirectional Unicode text that may be interpreted or compiled differently than what appears below. To review, open the file in an editor that reveals hidden Unicode characters.
Learn more about bidirectional Unicode characters
This file contains bidirectional Unicode text that may be interpreted or compiled differently than what appears below. To review, open the file in an editor that reveals hidden Unicode characters.
Learn more about bidirectional Unicode characters
Some generated files are not rendered by default. Learn more about how customized files appear on GitHub.
Oops, something went wrong.
Some generated files are not rendered by default. Learn more about how customized files appear on GitHub.
Oops, something went wrong.
This file contains bidirectional Unicode text that may be interpreted or compiled differently than what appears below. To review, open the file in an editor that reveals hidden Unicode characters.
Learn more about bidirectional Unicode characters
Original file line number | Diff line number | Diff line change |
---|---|---|
@@ -0,0 +1,258 @@ | ||
--- | ||
title: "Customized Sequential Design for Bayesian Optimization" | ||
output: rmarkdown::html_vignette | ||
bibliography: references.bib | ||
description: > | ||
Customized sequential design to implement Bayesian optimization for the Shubert function. | ||
vignette: > | ||
%\VignetteIndexEntry{Customized Sequential Design for Bayesian Optimization} | ||
%\VignetteEngine{knitr::rmarkdown} | ||
%\VignetteEncoding{UTF-8} | ||
--- | ||
|
||
```{r setup, include = FALSE} | ||
knitr::opts_chunk$set( | ||
echo = TRUE, | ||
eval = FALSE | ||
) | ||
``` | ||
|
||
This vignette demonstrates how to utilize and repurpose my favorite `design()` function in `dgpsi` to implement Bayesian optimization using the (D)GP surrogate models provided by the package. | ||
|
||
## Load the packages | ||
|
||
```{r} | ||
library(tidyverse) | ||
library(lhs) | ||
library(patchwork) | ||
library(ggplot2) | ||
library(dgpsi) | ||
``` | ||
|
||
## Define the target function | ||
|
||
We consider a version of the Shubert function [@surjanovic] defined over the domain $[0,1]^2$ as follows: | ||
|
||
```{r} | ||
shubert <- function(x) | ||
{ | ||
N <- nrow(x) | ||
x1 <- x[,1] * 4 - 2 | ||
x2 <- x[,2] * 4 - 2 | ||
ii <- c(1:5) | ||
y <- sapply(1:N, function(i) { | ||
sum(ii * cos((ii+1)*x1[i]+ii)) * sum(ii * cos((ii+1)*x2[i]+ii)) | ||
}) | ||
y <- matrix(y, ncol = 1) | ||
return(y) | ||
} | ||
``` | ||
|
||
To pass the Shubert function to `design()`, we defined it such that both its input `x` and output are matrices. Next, we generate the contour of the Shubert function: | ||
|
||
```{r} | ||
x1 <- seq(0, 1, length.out = 200) | ||
x2 <- seq(0, 1, length.out = 200) | ||
dat <- expand_grid(x1 = x1, x2 = x2) | ||
dat <- mutate(dat, f = shubert(cbind(x1, x2))) | ||
ggplot(dat, aes(x1, x2, fill = f)) + geom_tile() + | ||
scale_fill_continuous(type = "viridis") | ||
``` | ||
|
||
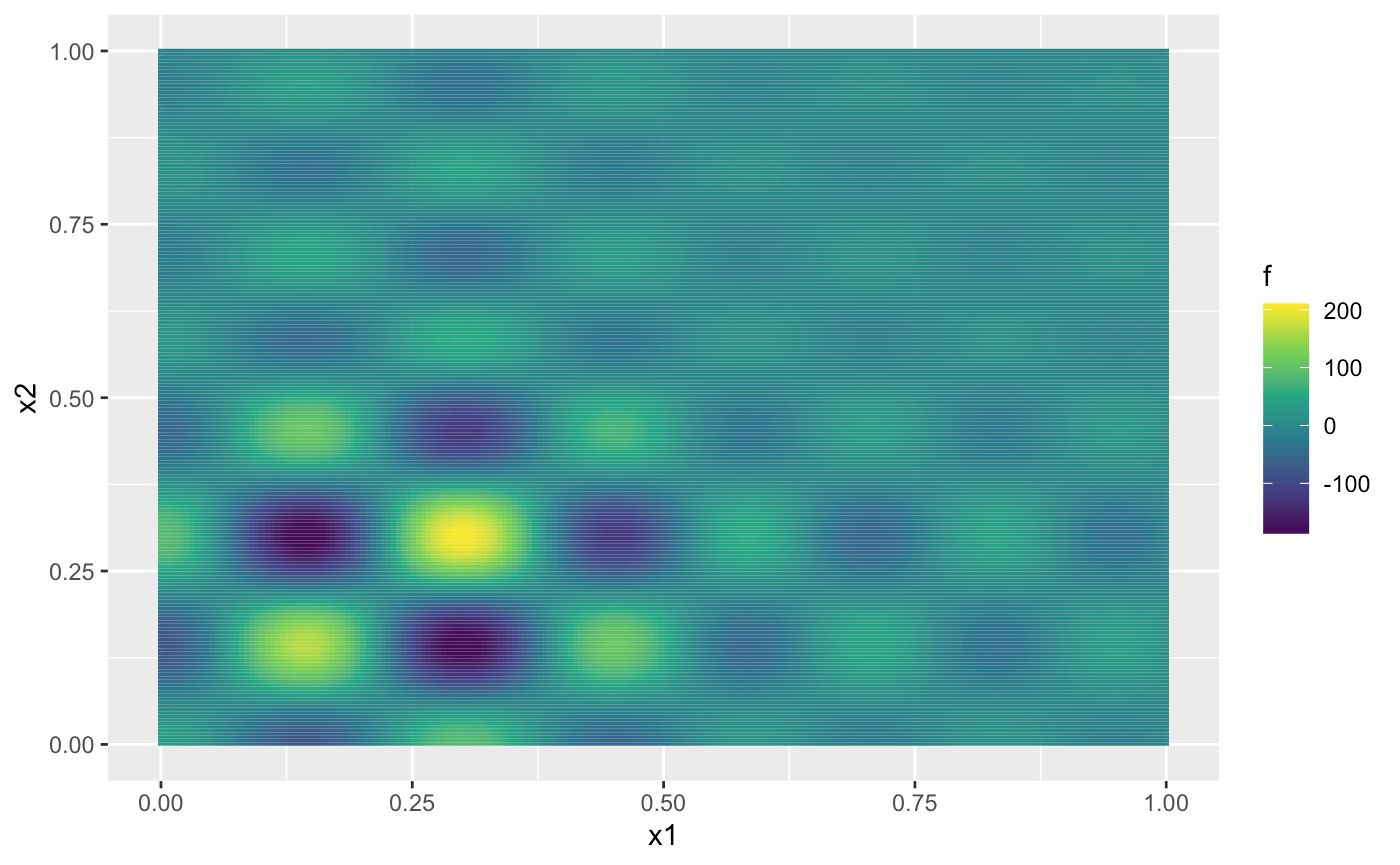{width=100%} | ||
|
||
From the figure above, we can observe that the Shubert function has several local minima and two global minima located at $(0.1437, 0.2999)$ and $(0.2999, 0.1437)$, both with values around $-186.7309$. In the remainder of this vignette, we will use Expected Improvement (EI) as the acquisition function to identify the global minima. | ||
|
||
## Initial emulator construction | ||
|
||
To ensure the reproducibility of this vignette, we set a seed using the `set_seed()` function from the package: | ||
|
||
```{r} | ||
set_seed(99) | ||
``` | ||
|
||
Next, we generate an initial design of 20 points using the maximin Latin hypercube sampler: | ||
|
||
```{r} | ||
X <- maximinLHS(20, 2) | ||
Y <- shubert(X) | ||
``` | ||
|
||
to construct the initial DGP surrogate model of the Shubert function: | ||
|
||
```{r} | ||
m <- dgp(X, Y, name = 'matern2.5') | ||
``` | ||
|
||
``` | ||
## Auto-generating a 2-layered DGP structure ... done | ||
## Initializing the DGP emulator ... done | ||
## Training the DGP emulator: | ||
## Iteration 500: Layer 2: 100%|██████████| 500/500 [00:02<00:00, 249.19it/s] | ||
## Imputing ... done | ||
``` | ||
|
||
## Bayesian optimization using Expected Improvement (EI) | ||
|
||
To begin Bayesian optimization with `design()`, we first need to define the Expected Improvement (EI) acquisition function, adhering to the rules specified for the `method` argument in `design()`: | ||
|
||
```{r} | ||
ei <- function(object, limits, n_starts = 10, ...) { | ||
# Expected Improvement (EI) function | ||
ei_function <- function(x) { | ||
x <- matrix(x, nrow = 1) | ||
# Extract mean and variance from the 'object' | ||
pred <- predict(object, x, ...)$results | ||
mu <- pred$mean | ||
sigma <- sqrt(pred$var) | ||
# Handle numerical precision issues with very small sigma values | ||
sigma[sigma < 1e-10] <- 1e-10 | ||
# Best observed minimum value | ||
best_value <- min(object$data$Y) | ||
# Calculate improvement | ||
improvement <- best_value - mu | ||
# Calculate Expected Improvement (EI) | ||
z <- improvement / sigma | ||
ei <- improvement * pnorm(z) + sigma * dnorm(z) | ||
ei <- ifelse(ei < 0, 0, ei) # Ensure non-negative EI | ||
return(-ei) # Return negative EI because `optim()` minimizes by default | ||
} | ||
# Number of input dimensions | ||
num_dims <- nrow(limits) | ||
# Generate initial points using Latin Hypercube Sampling (LHS) | ||
lhd_samples <- maximinLHS(n_starts, num_dims) | ||
lhd_points <- sapply(1:num_dims, function(i) { | ||
limits[i, 1] + lhd_samples[, i] * (limits[i, 2] - limits[i, 1]) | ||
}) | ||
# Perform optimization with multiple starts using `optim()` | ||
results <- lapply(1:n_starts, function(i) { | ||
optim( | ||
par = lhd_points[i, ], | ||
fn = ei_function, | ||
method = "L-BFGS-B", | ||
lower = limits[, 1], | ||
upper = limits[, 2] | ||
) | ||
}) | ||
# Find the result with the minimum (most negative) EI | ||
best_result <- results[[which.min(sapply(results, `[[`, "value"))]] | ||
# Return the next best point `x` as a single-row matrix | ||
return(matrix(best_result$par, nrow = 1)) | ||
} | ||
``` | ||
|
||
The `ei` function defined above takes the emulator as its first argument, `object`, along with the additional arguments: `limits`, a two-column matrix where the first column specifies the lower bounds and the second column specifies the upper bounds of the input dimensions, and `n_starts`, which defines the number of multi-start points used to search for the location corresponding to the minimum (most negative) EI. You can, of course, replace `ei` with your own acquisition function, provided it adheres to the rules of the `method` argument in `design()` (see `?design` for details). | ||
|
||
To track the identified minimum value of the Shubert function during Bayesian optimization, we define a monitor function, `opt_monitor`, to be passed to the `eval` argument of `design()`: | ||
|
||
```{r} | ||
opt_monitor <- function(object) { | ||
return(min(object$data$Y)) | ||
} | ||
``` | ||
|
||
The domain of the input parameters for our Bayesian optimization, within which the global minima are searched, is defined as $[0,1]^2$: | ||
|
||
```{r} | ||
lim <- rbind(c(0, 1), c(0, 1)) | ||
``` | ||
|
||
With all the ingredients ready, we can now run the Bayesian optimization using `design()` with 80 iterations: | ||
|
||
```{r} | ||
m <- design(m, N = 80, limits = lim, f = shubert, method = ei, eval = opt_monitor) | ||
``` | ||
|
||
``` | ||
## Initializing ... done | ||
## * Metric: -125.203479 | ||
## Iteration 1: | ||
## - Locating ... done | ||
## * Next design point: 0.352375 0.142570 | ||
## - Updating and re-fitting ... done | ||
## - Validating ... done | ||
## * Metric: -125.203479 | ||
## | ||
## ... | ||
## | ||
## Iteration 6: | ||
## - Locating ... done | ||
## * Next design point: 0.299331 0.125606 | ||
## - Updating and re-fitting ... done | ||
## - Transiting to a GP emulator ... done | ||
## - Validating ... done | ||
## * Metric: -186.039545 | ||
## | ||
## ... | ||
## | ||
## Iteration 80: | ||
## - Locating ... done | ||
## * Next design point: 0.907645 0.000000 | ||
## - Updating and re-fitting ... done | ||
## - Validating ... done | ||
## * Metric: -186.728582 | ||
``` | ||
|
||
During the Bayesian optimization, our dynamic pruning mechanism transitioned the DGP emulator to a GP emulator at iteration 6, leading to faster optimizations in the remaining iterations while maintaining the emulator's quality. After 80 iterations, we identified a minimum of the Shubert function with a value of $-186.7286$, which is very close to the global minimum of $-186.7309$. We can inspect the progress of the minima search conducted by `design()` by applying `draw()` to `m`: | ||
|
||
```{r} | ||
draw(m) + | ||
plot_annotation(title = 'Bayesian Optimization Tracker') + | ||
labs(y = "Minimum Value") + | ||
# Add a horizontal line to represent the global minimum for benchmarking | ||
geom_hline( | ||
aes(yintercept = y, linetype = "Global Minimum"), # Global minimum | ||
data = data.frame(y = -186.7309), | ||
color = "#E31A1C", | ||
size = 0.5 | ||
) + | ||
scale_linetype_manual( | ||
values = c("Global Minimum" = "dashed"), | ||
name = "" # Remove the legend title | ||
) | ||
``` | ||
|
||
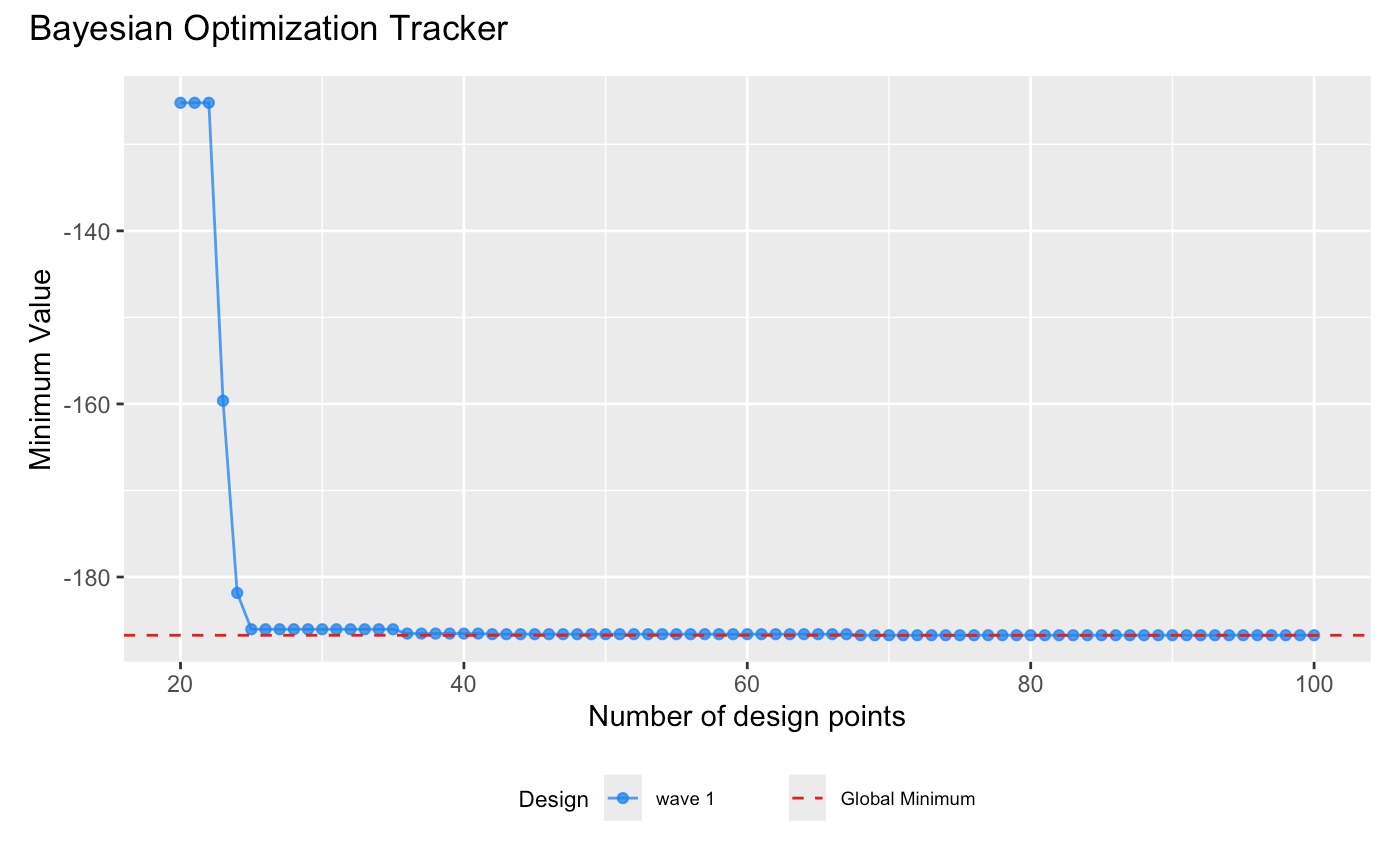{width=100%} | ||
|
||
The figure above shows that Bayesian optimization using our (D)GP emulator quickly identifies a low value of the Shubert function within 5 iterations. Notably, the lowest value, $-186.7286$, was achieved by `design()` after 48 iterations. | ||
|
||
We can also inspect the locations identified during the process by applying `draw()` to `m` with `type = 'design'`: | ||
|
||
```{r} | ||
draw(m, type = 'design') + | ||
plot_annotation(title = 'Bayesian Optimization Design') + | ||
# Highlight the global minima on the design plot | ||
geom_point( | ||
data = data.frame( | ||
.panel_x = c("X1", "X2"), # x labels of panels | ||
.panel_y = c("X2", "X1"), # y labels of panels | ||
X1 = c(0.1437, 0.2999), # X1 values for the global minima | ||
X2 = c(0.2999, 0.1437) # X2 values for the global minima | ||
), | ||
aes(x = .panel_x, y = .panel_y), | ||
size = 1.25, | ||
shape = 6, | ||
color = "#E31A1C" | ||
) | ||
``` | ||
|
||
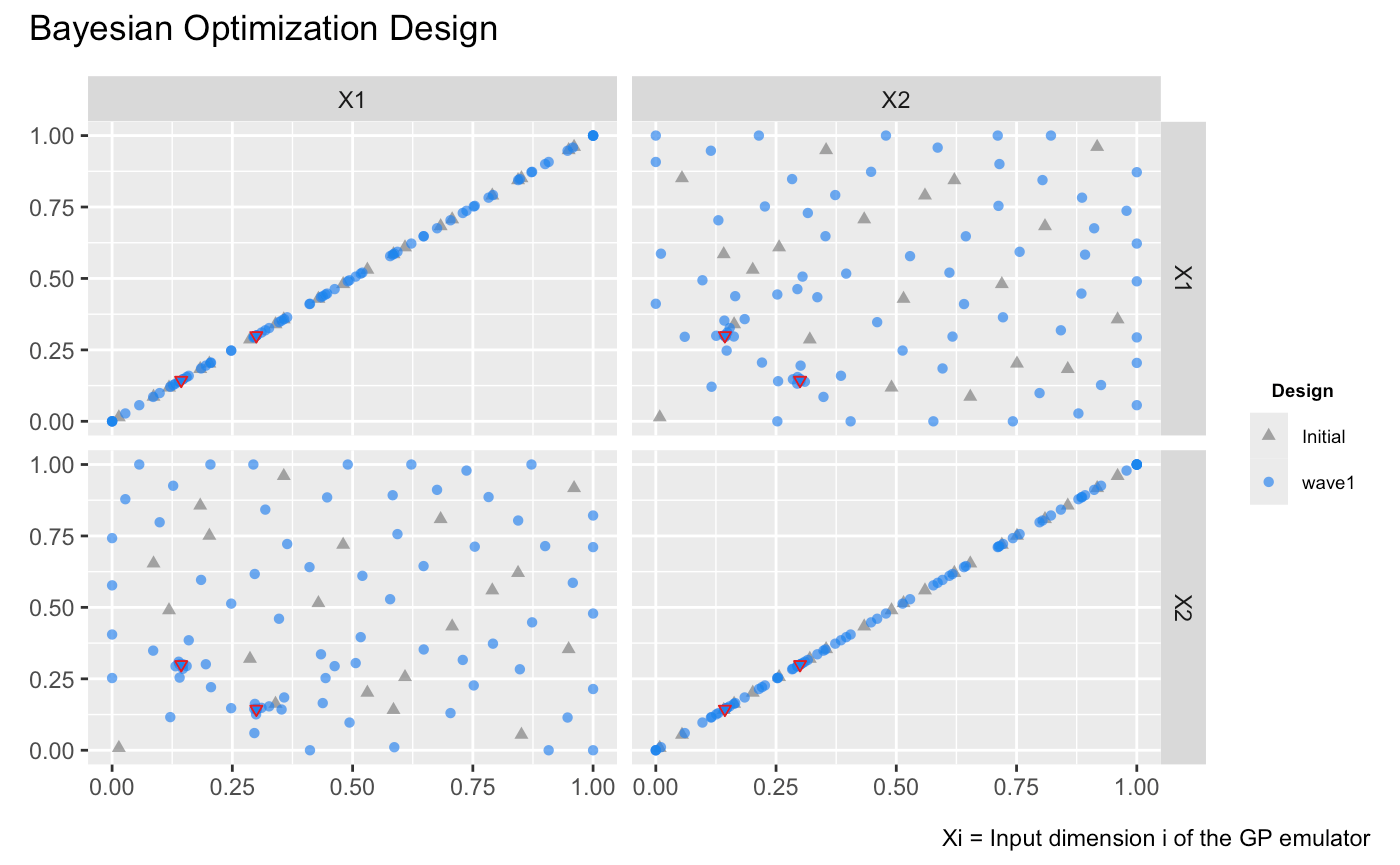{width=100%} | ||
|
||
The figure above illustrates that Bayesian optimization successfully identifies the regions around the two global minima, represented as red inverted triangles, by adding design points concentrated near these two locations. | ||
|
||
### References | ||
|
||
|
This file contains bidirectional Unicode text that may be interpreted or compiled differently than what appears below. To review, open the file in an editor that reveals hidden Unicode characters.
Learn more about bidirectional Unicode characters
Loading
Sorry, something went wrong. Reload?
Sorry, we cannot display this file.
Sorry, this file is invalid so it cannot be displayed.
Loading
Sorry, something went wrong. Reload?
Sorry, we cannot display this file.
Sorry, this file is invalid so it cannot be displayed.
Loading
Sorry, something went wrong. Reload?
Sorry, we cannot display this file.
Sorry, this file is invalid so it cannot be displayed.
This file contains bidirectional Unicode text that may be interpreted or compiled differently than what appears below. To review, open the file in an editor that reveals hidden Unicode characters.
Learn more about bidirectional Unicode characters