Commit
This commit does not belong to any branch on this repository, and may belong to a fork outside of the repository.
profiling package and results for pandas extensions (#11)
# Pull request description ## Branch summary The below package was used to generate the following performance results for the Ohio-Pandas integration extensions to `DataFrame` – `pg_copy_to` & `pg_copy_from`. ## Results summary In general, _all_ solutions involving the Ohio extensions require significantly less RAM; and, (except for the `buffer_size=1` case, which is no longer the default), Ohio extensions are also significantly faster. Unlike Pandas built-in methods `to_sql` and `read_sql`, doing a custom PostgreSQL `COPY` via `io.StringIO` *is* competitive, but still significantly slower and more RAM-intensive than Ohio extensions. (And Ohio extensions have the added benefit of being one-liners, simple method invocations, and "built-in" to `DataFrame`, once the integration is loaded.) ## Profiling The profiling package, `prof`, is executable as a package, (like a module): $ python -m prof … I added a management command wrapper largely for added documentation and cleanliness: $ manage profile … Generally, I used variations of the following command: $ time manage profile - --random --count 3 --plot ./plots/ ../dirtyduck/risks_aggregation.csv | tee profile-$(date +%s).txt *I.e.*: * Profile everything 3 times * Plot averages with error bars * Use some dirtyduck data – (it doesn't really matter what the CSV is, but it should be big, and the package was tested against something like this, with lots of floats and ints, and one as_of_dates column) * It's probably best to randomize the order in which things are profiled * It takes a long time to run, so GNU `time` reports on that. * Store console (debugging) output in a file. ## Results These take a really long time to run, and so while you could just run it as above, and get plots for each group, I generally filtered down to the one group of the two I was trying to test. ### Copy from DataFrame to database table $ time manage profile - --tag-filter "to database" --random --count 3 --plot ./plots/ ../dirtyduck/risks_aggregation.csv | tee profile-$(date +%s).txt 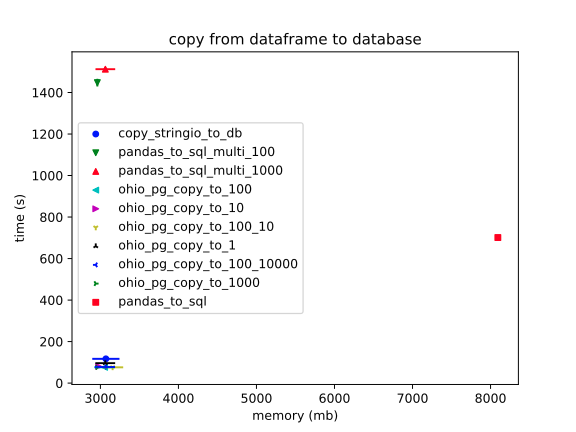 Basically, `to_sql` is a lost cause, (even playing with the `multi` parameter). However, the `StringIO` method comes close; and, it's unclear how close, at this scale. So, ignoring `to_sql`: $ time manage profile - --tag-filter "to database" --filter "^(copy|ohio)" --random --count 3 --plot ./plots/ ../dirtyduck/risks_aggregation.csv | tee profile-$(date +%s).txt 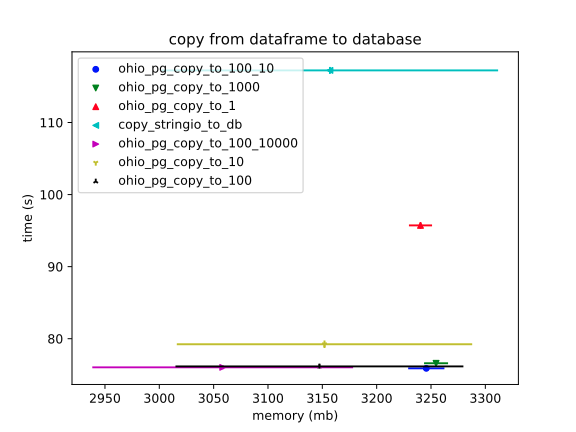 Now we can see more clearly what was apparent in the previous plot: the `StringIO` version is significantly slower. (This makes sense, since it has to encode _all_ the data into a string before beginning to `COPY` it into the database.) The error bars on RAM usage are a mess, however. It doesn't help that I'm comparing a ton of different configurations of Ohio, (including unreleased ones, which this profiling has almost certainly proven to be unnecessary). These RAM usage measurements were tough to make seem "true." Running all profilers, by default, in a subprocess made a huge difference; but, for whatever reason, you can still get a wide variance – perhaps because I'm just running these on my laptop, and even at three trials, a weird one can throw things off. In conclusion, I'd say that Ohio – especially with the current default configuration – does fair better on RAM usage, as well. And, that's just with this particular dataset. Unlike the `StringIO` version, the overhead of Ohio's solution shouldn't increase linearly. ### Copy from database table to DataFrame $ time manage profile - --tag-filter "to dataframe" --random --count 3 --plot ./plots/ ../dirtyduck/risks_aggregation.csv | tee profile-$(date +%s).txt 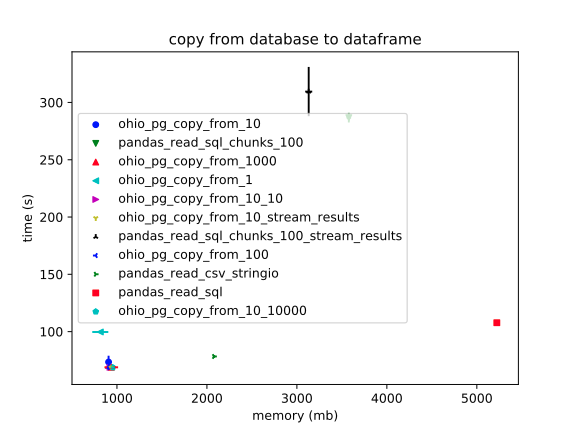 Pandas – `read_sql` – is again a huge outlier in slowness and RAM usage. $ time manage profile - -f "(ohio|stringio)" --tag-filter "to dataframe" --random --count 3 --plot ./plots/ ../dirtyduck/risks_aggregation.csv | tee profile-$(date +%s).txt 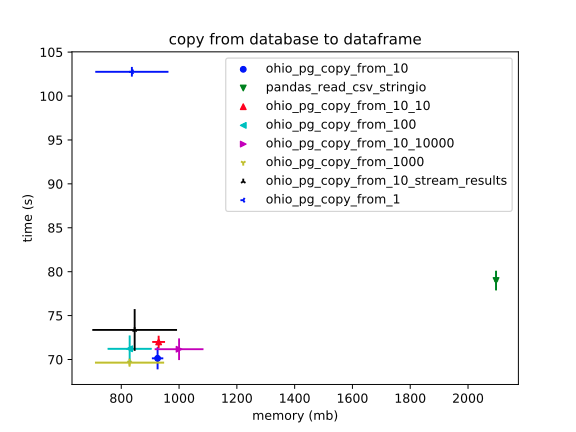 Unlike in the previous context, here `StringIO` does _much_ worse on RAM. And, again, it's significantly slower, (if not tremendously so). The Ohio solutions all do about the same, (and it's unclear which of them is definitely best). --- All that said, I'm happy to run more trials. And, with this package, others are welcome to do the same! 😸 # Pull request addendum All right I have: * moved some experimental stuff into dependent branch [experiment/profiling](profiling...experiment/profiling) * cleaned up some utility stuff and added missing doc strings * replaced custom `histogram` with `collections.Counter` * added a profiler of `DataFrame > StringIO > COPY` that goes through a `DataFrame` accessor, such that we're comparing apples-to-apples The results of the latter are interesting. 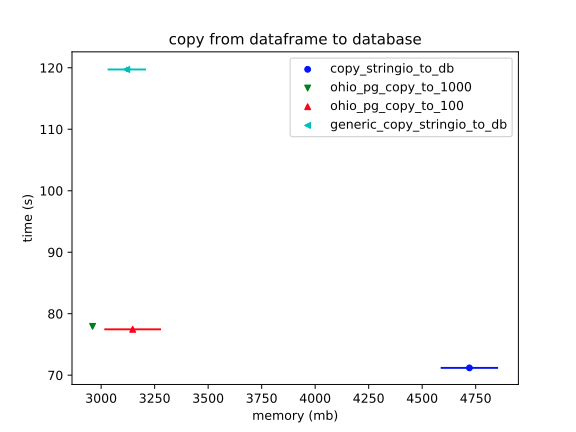 As we saw before, the "generic" version, which uses `df.to_csv(string_io)`, is *way* slow. It has similar RAM usage; but, that's misleading, since there might be some weird (and/or bad) RAM usage going on when Pandas initiates the extension. The novelty of the new profiler, which goes through a Pandas extension – `df.stringio_copy_to` – is that it should get no advantages with RAM. And it's ludicrous how much RAM it does use. (And, again, we can expect this to increase as the payload scales.) What's interesting is that the StringIO accessor is as *fast* as it is. It's just using `csv.writer`. So … 1. `DataFrame.to_csv(StringIO)` might be _terrible_, (at least without special configuration). 2. We're still gaining what we set out to gain from `pg_copy_to` – low, stable RAM usage, and *huge* a speed increase (thanks to `COPY`). The speed differential between `pg_copy_to` and the `StringIO` version isn't huge, (and the former is hugely preferable for its RAM usage). **But**, for future speed improvement, we might consider things like replacing `PipeTextIO` in `pg_copy_to` with another `ohio` utility, (as noted in that method's comments). (Or, an `asyncio` implementation of `PipeTextIO` _might_ help, as well.) # Pull request URL See: #11 # Change sets * initial profiling (ipython) scripting & local results * stand-alone profiling script & results for pg_copy_from * moved profile/ directory to prof/ to avoid conflict with built-in module `profile` * refactored profiling `prof` into executable package * added management command for profiling * completed profiling package and execution profile of pandas extensions * profilers for copying dataframe to database * profilers moved to subpackage * profiler group tagging * profilers executed in subprocess to ensure memory measurements * automatic plotting of memory & time results for each profiler group * randomize profiler order in group * run multiple trials * filter by profiler function name and/or by group tag * removed in-development artifacts (txt, yaml, png) * made management command wrapper handle arguments and errors correctly * updated profiling package requirements * removed profilers of experimental feature `iter_size` * functional decorator for `Wrapper` class * use built-in `collections.Counter` in place of custom `histogram` * ``__doc__`` for ``tool`` & ``util`` * profiler for StringIO -> COPY via DataFrame accessor This profiler permits a proper comparision to pg_copy_to, (and exposes potential opportunities for improvement).
- Loading branch information