You signed in with another tab or window. Reload to refresh your session.You signed out in another tab or window. Reload to refresh your session.You switched accounts on another tab or window. Reload to refresh your session.Dismiss alert
Hi,thanks for your great work.
I am a newbie on Deep Learning,i have some question about the paper.
In your paper ,you said that : We use the 1-cycle policy for the learning rate with max_lr = 3.5 × 10−4 , linear warm-up from max_lr/25 to max lr for the first 30% of iterations followed by cosine annealing to max_lr/75.
and the code in your work is:
I find that the learning rate is not increasing with the linear function but the cosine-like funtion.
I wrote a code following the setting in your work,and visualized the learning rate change.Here is the code and the result respectively
import torch
from torch.optim.lr_scheduler import CosineAnnealingLR, CosineAnnealingWarmRestarts,StepLR, OneCycleLR
import torch.nn as nn
from torchvision.models import resnet18
import matplotlib.pyplot as plt
if __name__ == '__main__':
model = resnet18(pretrained=False)
optimizer = torch.optim.SGD(model.parameters(), lr=0.1)
mode = 'OneCycleLR'
max_epoch = 100
iters = 200
scheduler = OneCycleLR(optimizer, max_lr=10, steps_per_epoch=iters, epochs=max_epoch, pct_start=0.3,
div_factor=10,final_div_factor=100,verbose=True,three_phase =False,
anneal_strategy='cos',
cycle_momentum = True,base_momentum=0.85, max_momentum=0.95)
plt.figure()
cur_lr_list = []
for epoch in range(max_epoch):
for batch in range(iters):
optimizer.step()
scheduler.step()
cur_lr = optimizer.param_groups[-1]['lr']
cur_lr_list.append(cur_lr)
# print('Cur lr:', cur_lr)
x_list = list(range(len(cur_lr_list)))
plt.plot(x_list, cur_lr_list)
plt.show()
I am so confused about this question,could you tell me the reason?Thanks.
The text was updated successfully, but these errors were encountered:
Hi,thanks for your great work.
I am a newbie on Deep Learning,i have some question about the paper.
In your paper ,you said that :
We use the 1-cycle policy for the learning rate with max_lr = 3.5 × 10−4 , linear warm-up from max_lr/25 to max lr for the first 30% of iterations followed by cosine annealing to max_lr/75.
and the code in your work is:
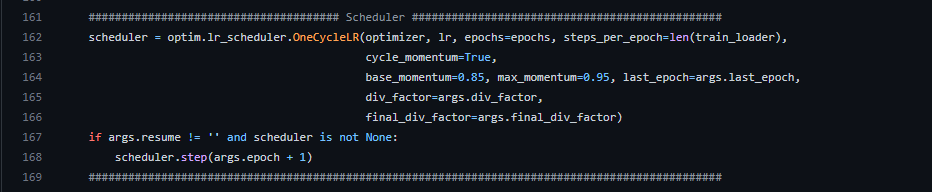
I find that the learning rate is not increasing with the linear function but the cosine-like funtion.
I wrote a code following the setting in your work,and visualized the learning rate change.Here is the code and the result respectively
I am so confused about this question,could you tell me the reason?Thanks.
The text was updated successfully, but these errors were encountered: