From 436d48818d0c2e48fcb2ceb1fcb1fc411e2e192c Mon Sep 17 00:00:00 2001
From: =?UTF-8?q?Haian=20Huang=28=E6=B7=B1=E5=BA=A6=E7=9C=B8=29?=
<1286304229@qq.com>
Date: Fri, 5 Jan 2024 14:04:08 +0800
Subject: [PATCH] Bump version to 3.3.0 (#11338)
---
README.md | 44 ++---------------
README_zh-CN.md | 45 +++--------------
configs/mm_grounding_dino/README.md | 12 ++++-
configs/mm_grounding_dino/dataset_prepare.md | 2 +-
.../dataset_prepare_zh-CN.md | 4 +-
configs/mm_grounding_dino/usage.md | 7 +--
configs/mm_grounding_dino/usage_zh-CN.md | 6 +--
docker/serve/Dockerfile | 2 +-
docker/serve_cn/Dockerfile | 2 +-
docs/en/notes/changelog.md | 30 +++++++++++-
docs/en/notes/faq.md | 1 +
docs/zh_cn/notes/faq.md | 1 +
mmdet/version.py | 2 +-
tools/dataset_converters/grit_processing.py | 49 ++++++-------------
14 files changed, 82 insertions(+), 125 deletions(-)
diff --git a/README.md b/README.md
index edeac51017e..15f71dad5fb 100644
--- a/README.md
+++ b/README.md
@@ -103,50 +103,16 @@ Apart from MMDetection, we also released [MMEngine](https://github.com/open-mmla
### Highlight
-**v3.2.0** was released in 12/10/2023:
+**v3.3.0** was released in 5/1/2024:
-**1. Detection Transformer SOTA Model Collection**
-(1) Supported four updated and stronger SOTA Transformer models: [DDQ](configs/ddq/README.md), [CO-DETR](projects/CO-DETR/README.md), [AlignDETR](projects/AlignDETR/README.md), and [H-DINO](projects/HDINO/README.md).
-(2) Based on CO-DETR, MMDet released a model with a COCO performance of 64.1 mAP.
-(3) Algorithms such as DINO support `AMP/Checkpoint/FrozenBN`, which can effectively reduce memory usage.
+**[MM-Grounding-DINO: An Open and Comprehensive Pipeline for Unified Object Grounding and Detection](https://arxiv.org/abs/2401.02361)**
-**2. [Comprehensive Performance Comparison between CNN and Transformer](projects/RF100-Benchmark/README.md)**
-RF100 consists of a dataset collection of 100 real-world datasets, including 7 domains. It can be used to assess the performance differences of Transformer models like DINO and CNN-based algorithms under different scenarios and data volumes. Users can utilize this benchmark to quickly evaluate the robustness of their algorithms in various scenarios.
+Grounding DINO is a grounding pre-training model that unifies 2d open vocabulary object detection and phrase grounding, with wide applications. However, its training part has not been open sourced. Therefore, we propose MM-Grounding-DINO, which not only serves as an open source replication version of Grounding DINO, but also achieves significant performance improvement based on reconstructed data types, exploring different dataset combinations and initialization strategies. Moreover, we conduct evaluations from multiple dimensions, including OOD, REC, Phrase Grounding, OVD, and Fine-tune, to fully excavate the advantages and disadvantages of Grounding pre-training, hoping to provide inspiration for future work.
-
-

-
-
-**3. Support for [GLIP](configs/glip/README.md) and [Grounding DINO](configs/grounding_dino/README.md) fine-tuning, the only algorithm library that supports Grounding DINO fine-tuning**
-The Grounding DINO algorithm in MMDet is the only library that supports fine-tuning. Its performance is one point higher than the official version, and of course, GLIP also outperforms the official version.
-We also provide a detailed process for training and evaluating Grounding DINO on custom datasets. Everyone is welcome to give it a try.
-
-| Model | Backbone | Style | COCO mAP | Official COCO mAP |
-| :----------------: | :------: | :-------: | :--------: | :---------------: |
-| Grounding DINO-T | Swin-T | Zero-shot | 48.5 | 48.4 |
-| Grounding DINO-T | Swin-T | Finetune | 58.1(+0.9) | 57.2 |
-| Grounding DINO-B | Swin-B | Zero-shot | 56.9 | 56.7 |
-| Grounding DINO-B | Swin-B | Finetune | 59.7 | |
-| Grounding DINO-R50 | R50 | Scratch | 48.9(+0.8) | 48.1 |
-
-**4. Support for the open-vocabulary detection algorithm [Detic](projects/Detic_new/README.md) and multi-dataset joint training.**
-**5. Training detection models using [FSDP and DeepSpeed](projects/example_largemodel/README.md).**
-
-| ID | AMP | GC of Backbone | GC of Encoder | FSDP | Peak Mem (GB) | Iter Time (s) |
-| :-: | :-: | :------------: | :-----------: | :--: | :-----------: | :-----------: |
-| 1 | | | | | 49 (A100) | 0.9 |
-| 2 | √ | | | | 39 (A100) | 1.2 |
-| 3 | | √ | | | 33 (A100) | 1.1 |
-| 4 | √ | √ | | | 25 (A100) | 1.3 |
-| 5 | | √ | √ | | 18 | 2.2 |
-| 6 | √ | √ | √ | | 13 | 1.6 |
-| 7 | | √ | √ | √ | 14 | 2.9 |
-| 8 | √ | √ | √ | √ | 8.5 | 2.4 |
-
-**6. Support for the [V3Det](configs/v3det/README.md) dataset, a large-scale detection dataset with over 13,000 categories.**
+code: [mm_grounding_dino/README.md](configs/mm_grounding_dino/README.md)
-
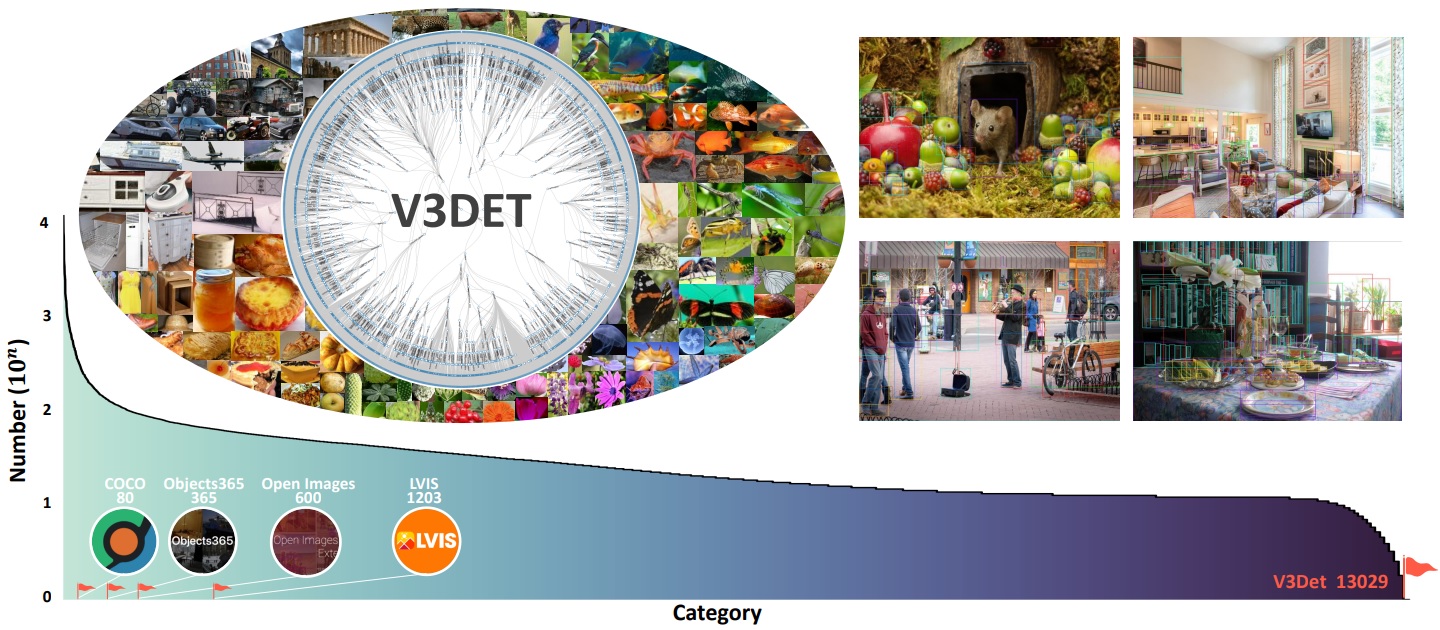
+
We are excited to announce our latest work on real-time object recognition tasks, **RTMDet**, a family of fully convolutional single-stage detectors. RTMDet not only achieves the best parameter-accuracy trade-off on object detection from tiny to extra-large model sizes but also obtains new state-of-the-art performance on instance segmentation and rotated object detection tasks. Details can be found in the [technical report](https://arxiv.org/abs/2212.07784). Pre-trained models are [here](configs/rtmdet).
diff --git a/README_zh-CN.md b/README_zh-CN.md
index ccf1cbf0082..885d1f22617 100644
--- a/README_zh-CN.md
+++ b/README_zh-CN.md
@@ -102,51 +102,18 @@ MMDetection 是一个基于 PyTorch 的目标检测开源工具箱。它是 [Ope
### 亮点
-**v3.2.0** 版本已经在 2023.10.12 发布:
+**v3.3.0** 版本已经在 2024.1.5 发布:
-**1. 检测 Transformer SOTA 模型大合集**
-(1) 支持了 [DDQ](configs/ddq/README.md)、[CO-DETR](projects/CO-DETR/README.md)、[AlignDETR](projects/AlignDETR/README.md) 和 [H-DINO](projects/HDINO/README.md) 4 个更新更强的 SOTA Transformer 模型
-(2) 基于 CO-DETR, MMDet 中发布了 COCO 性能为 64.1 mAP 的模型
-(3) DINO 等算法支持 AMP/Checkpoint/FrozenBN,可以有效降低显存
+**MM-Grounding-DINO: 轻松涨点,数据到评测全面开源**
-**2. [提供了全面的 CNN 和 Transformer 的性能对比](projects/RF100-Benchmark/README_zh-CN.md)**
-RF100 是由 100 个现实收集的数据集组成,包括 7 个域,可以验证 DINO 等 Transformer 模型和 CNN 类算法在不同场景不同数据量下的性能差异。用户可以用这个 Benchmark 快速验证自己的算法在不同场景下的鲁棒性。
+Grounding DINO 是一个统一了 2d 开放词汇目标检测和 Phrase Grounding 的检测预训练模型,应用广泛,但是其训练部分并未开源,为此提出了 MM-Grounding-DINO。其不仅作为 Grounding DINO 的开源复现版,MM-Grounding-DINO 基于重新构建的数据类型出发,在探索了不同数据集组合和初始化策略基础上实现了 Grounding DINO 的性能极大提升,并且从多个维度包括 OOD、REC、Phrase Grounding、OVD 和 Finetune 等方面进行评测,充分挖掘 Grounding 预训练优缺点,希望能为后续工作提供启发。
-
-

-
-
-**3. 支持了 [GLIP](configs/glip/README.md) 和 [Grounding DINO](configs/grounding_dino/README.md) 微调,全网唯一支持 Grounding DINO 微调**
-MMDet 中的 Grounding DINO 是全网唯一支持微调的算法库,且性能高于官方 1 个点,当然 GLIP 也比官方高。
-我们还提供了详细的 Grounding DINO 在自定义数据集上训练评估的流程,欢迎大家试用。
-
-| Model | Backbone | Style | COCO mAP | Official COCO mAP |
-| :----------------: | :------: | :-------: | :--------: | :---------------: |
-| Grounding DINO-T | Swin-T | Zero-shot | 48.5 | 48.4 |
-| Grounding DINO-T | Swin-T | Finetune | 58.1(+0.9) | 57.2 |
-| Grounding DINO-B | Swin-B | Zero-shot | 56.9 | 56.7 |
-| Grounding DINO-B | Swin-B | Finetune | 59.7 | |
-| Grounding DINO-R50 | R50 | Scratch | 48.9(+0.8) | 48.1 |
-
-**4. 支持开放词汇检测算法 [Detic](projects/Detic_new/README.md) 并提供多数据集联合训练可能**
-
-**5. 轻松使用 [FSDP 和 DeepSpeed 训练检测模型](projects/example_largemodel/README_zh-CN.md)**
-
-| ID | AMP | GC of Backbone | GC of Encoder | FSDP | Peak Mem (GB) | Iter Time (s) |
-| :-: | :-: | :------------: | :-----------: | :--: | :-----------: | :-----------: |
-| 1 | | | | | 49 (A100) | 0.9 |
-| 2 | √ | | | | 39 (A100) | 1.2 |
-| 3 | | √ | | | 33 (A100) | 1.1 |
-| 4 | √ | √ | | | 25 (A100) | 1.3 |
-| 5 | | √ | √ | | 18 | 2.2 |
-| 6 | √ | √ | √ | | 13 | 1.6 |
-| 7 | | √ | √ | √ | 14 | 2.9 |
-| 8 | √ | √ | √ | √ | 8.5 | 2.4 |
+arxiv 技术报告:https://arxiv.org/abs/2401.02361
-**6. 支持了 [V3Det](configs/v3det/README.md) 1.3w+ 类别的超大词汇检测数据集**
+代码地址: [mm_grounding_dino/README.md](configs/mm_grounding_dino/README.md)
-
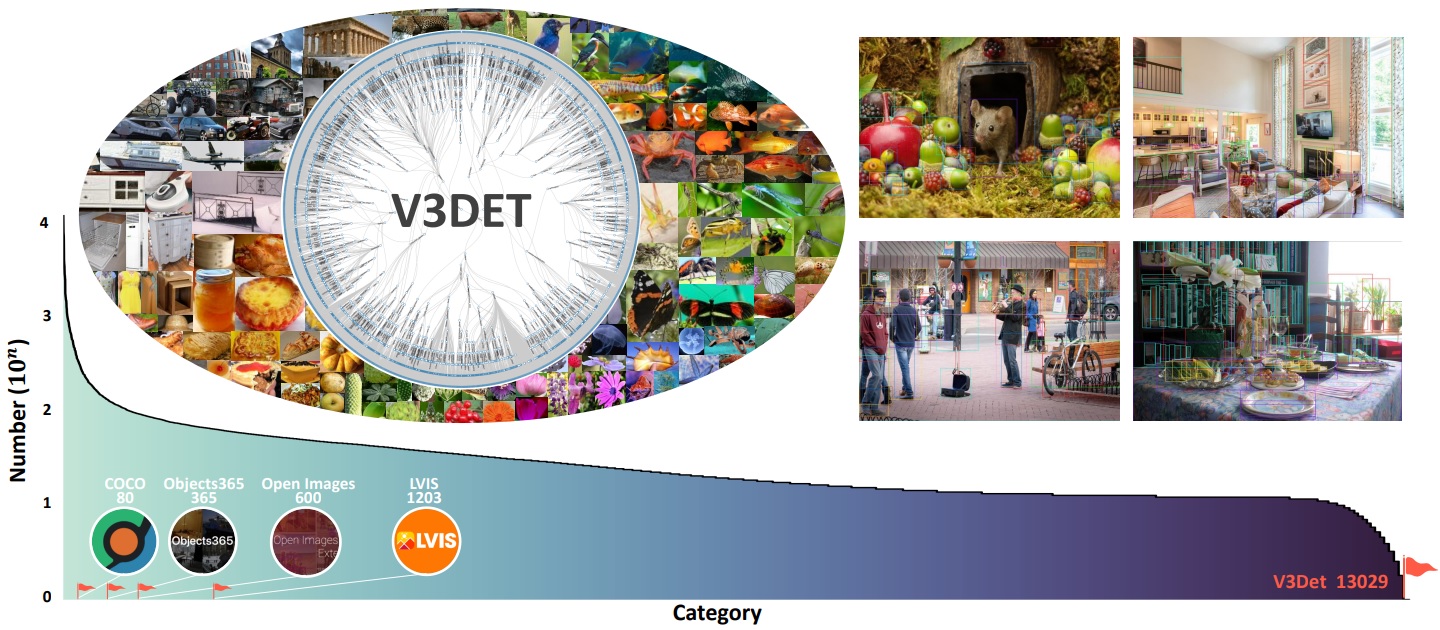
+
我们很高兴向大家介绍我们在实时目标识别任务方面的最新成果 RTMDet,包含了一系列的全卷积单阶段检测模型。 RTMDet 不仅在从 tiny 到 extra-large 尺寸的目标检测模型上实现了最佳的参数量和精度的平衡,而且在实时实例分割和旋转目标检测任务上取得了最先进的成果。 更多细节请参阅[技术报告](https://arxiv.org/abs/2212.07784)。 预训练模型可以在[这里](configs/rtmdet)找到。
diff --git a/configs/mm_grounding_dino/README.md b/configs/mm_grounding_dino/README.md
index eda2c1da5f1..bcc913446dc 100644
--- a/configs/mm_grounding_dino/README.md
+++ b/configs/mm_grounding_dino/README.md
@@ -1,10 +1,20 @@
# MM Grounding DINO
+> [An Open and Comprehensive Pipeline for Unified Object Grounding and Detection](https://arxiv.org/abs/2401.02361)
+
## Abstract
-TODO
+Grounding-DINO is a state-of-the-art open-set detection model that tackles multiple vision tasks including Open-Vocabulary Detection (OVD), Phrase Grounding (PG), and Referring Expression Comprehension (REC). Its effectiveness has led to its widespread adoption as a mainstream architecture for various downstream applications. However, despite its significance, the original Grounding-DINO model lacks comprehensive public technical details due to the unavailability of its training code. To bridge this gap, we present MM-Grounding-DINO, an open-source, comprehensive, and user-friendly baseline, which is built with the MMDetection toolbox. It adopts abundant vision datasets for pre-training and various detection and grounding datasets for fine-tuning. We give a comprehensive analysis of each reported result and detailed settings for reproduction. The extensive experiments on the benchmarks mentioned demonstrate that our MM-Grounding-DINO-Tiny outperforms the Grounding-DINO-Tiny baseline. We release all our models to the research community.
+
+
+
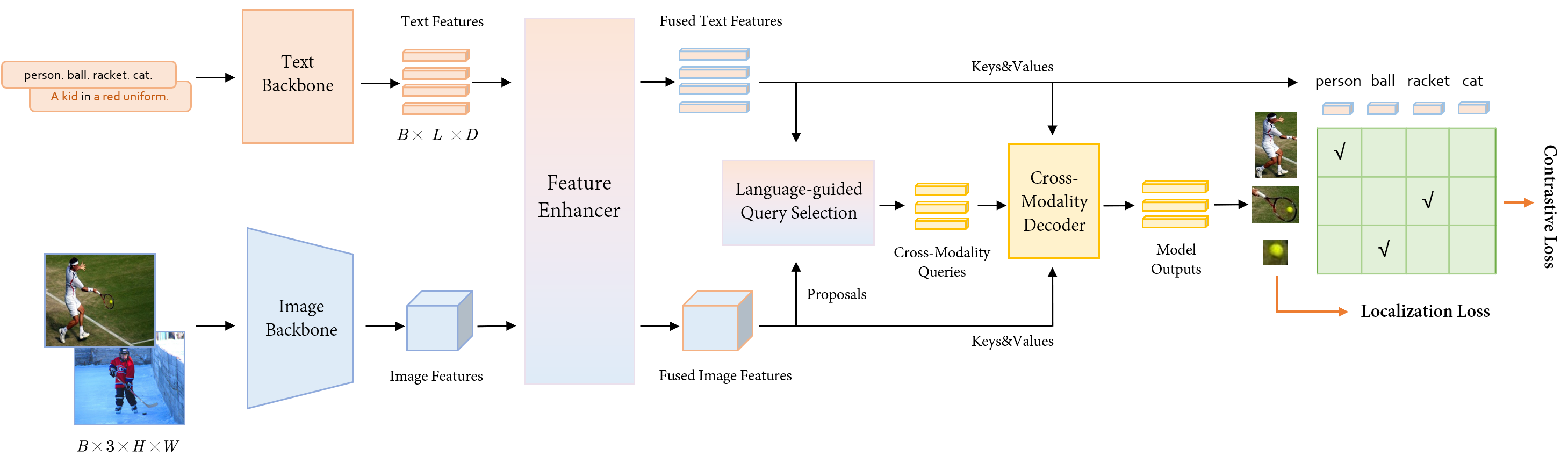
+
+
+
+

+
## Dataset Preparation
diff --git a/configs/mm_grounding_dino/dataset_prepare.md b/configs/mm_grounding_dino/dataset_prepare.md
index 160c4b98837..af60a8bf4bf 100644
--- a/configs/mm_grounding_dino/dataset_prepare.md
+++ b/configs/mm_grounding_dino/dataset_prepare.md
@@ -197,7 +197,7 @@ mmdetection
As for the GRIT dataset, you need to use [grit2odvg.py](../../tools/dataset_converters/grit2odvg.py) to convert it to the format of ODVG:
-```python
+```shell
python tools/dataset_converters/grit2odvg.py data/grit_processed/
```
diff --git a/configs/mm_grounding_dino/dataset_prepare_zh-CN.md b/configs/mm_grounding_dino/dataset_prepare_zh-CN.md
index 31647e91c5d..10520b02fe5 100644
--- a/configs/mm_grounding_dino/dataset_prepare_zh-CN.md
+++ b/configs/mm_grounding_dino/dataset_prepare_zh-CN.md
@@ -197,11 +197,11 @@ mmdetection
对于 GRIT 数据集,你需要使用 [grit2odvg.py](../../tools/dataset_converters/grit2odvg.py) 转化成需要的 ODVG 格式:
-```python
+```shell
python tools/dataset_converters/grit2odvg.py data/grit_processed/
```
-程序运行完成后会在 `data/grit_processed` 目录下创建 `grit20m_vg.json` 新文件,大概包含 9M 数据,完整结构如下:
+程序运行完成后会在 `data/grit_processed` 目录下创建 `grit20m_vg.json` 新文件,大概包含 9M 条数据,完整结构如下:
```text
mmdetection
diff --git a/configs/mm_grounding_dino/usage.md b/configs/mm_grounding_dino/usage.md
index f0773c8cf0e..123c6638cbe 100644
--- a/configs/mm_grounding_dino/usage.md
+++ b/configs/mm_grounding_dino/usage.md
@@ -133,14 +133,15 @@ python demo/image_demo.py images/fruit.jpg \
configs/mm_grounding_dino/grounding_dino_swin-t_pretrain_obj365.py \
--weights grounding_dino_swin-t_pretrain_obj365_goldg_grit9m_v3det_20231204_095047-b448804b.pth \
--texts 'The picture contains watermelon, flower, and a white bottle.' \
- --tokens-positive "[[[21,30]], [[45,59]]]" --pred-score-thr 0.12
+ --tokens-positive "[[[21,31]], [[45,59]]]" --pred-score-thr 0.12
```
-The noun phrase corresponding to positions 21-30 is `watermelon`, and the noun phrase corresponding to positions 45-59 is `a white bottle`.
+The noun phrase corresponding to positions 21-31 is `watermelon`, and the noun phrase corresponding to positions 45-59 is `a white bottle`.
-

+
+
**(4) Referential Expression Comprehension**
Referential expression understanding refers to the model automatically comprehending the referential expressions involved in a user's language description without the need for noun phrase extraction.
diff --git a/configs/mm_grounding_dino/usage_zh-CN.md b/configs/mm_grounding_dino/usage_zh-CN.md
index 0e5e1a766df..5f625ea6ca8 100644
--- a/configs/mm_grounding_dino/usage_zh-CN.md
+++ b/configs/mm_grounding_dino/usage_zh-CN.md
@@ -133,13 +133,13 @@ python demo/image_demo.py images/fruit.jpg \
configs/mm_grounding_dino/grounding_dino_swin-t_pretrain_obj365.py \
--weights grounding_dino_swin-t_pretrain_obj365_goldg_grit9m_v3det_20231204_095047-b448804b.pth \
--texts 'The picture contains watermelon, flower, and a white bottle.' \
- --tokens-positive "[[[21,30]], [[45,59]]]" --pred-score-thr 0.12
+ --tokens-positive "[[[21,31]], [[45,59]]]" --pred-score-thr 0.12
```
-21,30 对应的名词短语为 `watermelon`,45,59 对应的名词短语为 `a white bottle`。
+21,31 对应的名词短语为 `watermelon`,45,59 对应的名词短语为 `a white bottle`。
-

+
**(4) 指代性表达式理解**
diff --git a/docker/serve/Dockerfile b/docker/serve/Dockerfile
index 872918972f0..aa307cf6963 100644
--- a/docker/serve/Dockerfile
+++ b/docker/serve/Dockerfile
@@ -4,7 +4,7 @@ ARG CUDNN="8"
FROM pytorch/pytorch:${PYTORCH}-cuda${CUDA}-cudnn${CUDNN}-devel
ARG MMCV="2.0.0rc4"
-ARG MMDET="3.2.0"
+ARG MMDET="3.3.0"
ENV PYTHONUNBUFFERED TRUE
diff --git a/docker/serve_cn/Dockerfile b/docker/serve_cn/Dockerfile
index 510906432b7..894e15dd714 100644
--- a/docker/serve_cn/Dockerfile
+++ b/docker/serve_cn/Dockerfile
@@ -4,7 +4,7 @@ ARG CUDNN="8"
FROM pytorch/pytorch:${PYTORCH}-cuda${CUDA}-cudnn${CUDNN}-devel
ARG MMCV="2.0.0rc4"
-ARG MMDET="3.2.0"
+ARG MMDET="3.3.0"
ENV PYTHONUNBUFFERED TRUE
diff --git a/docs/en/notes/changelog.md b/docs/en/notes/changelog.md
index 4d48a0a0d22..00ed8f1c1e4 100644
--- a/docs/en/notes/changelog.md
+++ b/docs/en/notes/changelog.md
@@ -1,6 +1,34 @@
# Changelog of v3.x
-## v3.1.0 (12/10/2023)
+## v3.3.0 (05/01/2024)
+
+### Highlights
+
+Grounding-DINO is a state-of-the-art open-set detection model that tackles multiple vision tasks including Open-Vocabulary Detection (OVD), Phrase Grounding (PG), and Referring Expression Comprehension (REC). Its effectiveness has led to its widespread adoption as a mainstream architecture for various downstream applications. However, despite its significance, the original Grounding-DINO model lacks comprehensive public technical details due to the unavailability of its training code. To bridge this gap, we present MM-Grounding-DINO, an open-source, comprehensive, and user-friendly baseline, which is built with the MMDetection toolbox. It adopts abundant vision datasets for pre-training and various detection and grounding datasets for fine-tuning. We give a comprehensive analysis of each reported result and detailed settings for reproduction. The extensive experiments on the benchmarks mentioned demonstrate that our MM-Grounding-DINO-Tiny outperforms the Grounding-DINO-Tiny baseline. We release all our models to the research community.
+
+### New Features
+
+- Add RTMDet Swin / ConvNeXt backbone and results (#11259)
+- Add `odinw` configs and evaluation results of `GLIP` (#11175)
+- Add optional score threshold option to `coco_error_analysis.py` (#11117)
+- Add new configs for `panoptic_fpn` (#11109)
+- Replace partially weighted download links with OpenXLab for the `Faster-RCNN` (#11173)
+
+### Bug Fixes
+
+- Fix `Grounding DINO` nan when class tokens exceeds 256 (#11066)
+- Fix the `CO-DETR` config files error (#11325)
+- Fix `CO-DETR` load_from url in config (#11220)
+- Fixed mask shape after Albu postprocess (#11280)
+- Fix bug in `convert_coco_format` and `youtubevis2coco` (#11251, #11086)
+
+### Contributors
+
+A total of 15 developers contributed to this release.
+
+Thank @adnan-mujagic, @Cycyes, @ilcopione, @returnL, @honeybadger78, @okotaku, @xushilin1, @keyhsw, @guyleaf, @Crescent-Saturn, @LRJKD, @aaronzs, @Divadi, @AwePhD, @hhaAndroid
+
+## v3.2.0 (12/10/2023)
### Highlights
diff --git a/docs/en/notes/faq.md b/docs/en/notes/faq.md
index 9e3c1a7852b..f1a176e4d04 100644
--- a/docs/en/notes/faq.md
+++ b/docs/en/notes/faq.md
@@ -47,6 +47,7 @@ Compatible MMDetection, MMEngine, and MMCV versions are shown as below. Please c
| MMDetection version | MMCV version | MMEngine version |
| :-----------------: | :---------------------: | :----------------------: |
| main | mmcv>=2.0.0, \<2.2.0 | mmengine>=0.7.1, \<1.0.0 |
+| 3.3.0 | mmcv>=2.0.0, \<2.2.0 | mmengine>=0.7.1, \<1.0.0 |
| 3.2.0 | mmcv>=2.0.0, \<2.2.0 | mmengine>=0.7.1, \<1.0.0 |
| 3.1.0 | mmcv>=2.0.0, \<2.1.0 | mmengine>=0.7.1, \<1.0.0 |
| 3.0.0 | mmcv>=2.0.0, \<2.1.0 | mmengine>=0.7.1, \<1.0.0 |
diff --git a/docs/zh_cn/notes/faq.md b/docs/zh_cn/notes/faq.md
index 8268bd11562..2b4237c7411 100644
--- a/docs/zh_cn/notes/faq.md
+++ b/docs/zh_cn/notes/faq.md
@@ -47,6 +47,7 @@ export DYNAMO_CACHE_SIZE_LIMIT = 4
| MMDetection 版本 | MMCV 版本 | MMEngine 版本 |
| :--------------: | :---------------------: | :----------------------: |
| main | mmcv>=2.0.0, \<2.2.0 | mmengine>=0.7.1, \<1.0.0 |
+ | 3.3.0 | mmcv>=2.0.0, \<2.2.0 | mmengine>=0.7.1, \<1.0.0 |
| 3.2.0 | mmcv>=2.0.0, \<2.2.0 | mmengine>=0.7.1, \<1.0.0 |
| 3.1.0 | mmcv>=2.0.0, \<2.1.0 | mmengine>=0.7.1, \<1.0.0 |
| 3.0.0 | mmcv>=2.0.0, \<2.1.0 | mmengine>=0.7.1, \<1.0.0 |
diff --git a/mmdet/version.py b/mmdet/version.py
index 38ce834e152..47989fc0a31 100644
--- a/mmdet/version.py
+++ b/mmdet/version.py
@@ -1,6 +1,6 @@
# Copyright (c) OpenMMLab. All rights reserved.
-__version__ = '3.2.0'
+__version__ = '3.3.0'
short_version = __version__
diff --git a/tools/dataset_converters/grit_processing.py b/tools/dataset_converters/grit_processing.py
index 923093ab4fc..ebf3791a80e 100644
--- a/tools/dataset_converters/grit_processing.py
+++ b/tools/dataset_converters/grit_processing.py
@@ -43,13 +43,8 @@ def count_download_image(download_json_dir, logger):
def tar_processing(tar_path, output_dir, logger):
- """解压tar文件到对应名字的文件夹,并提取所有的json combine后,删除其他保存图片."""
- # 创建文件夹并解压
filepath = untar(tar_path, logger)
- '''将所有json融合为一个json'''
- # 获取解压后目录下所有的.json文件
json_files = [f for f in os.listdir(filepath) if f.endswith('.json')]
- # 初始化一个空的列表来存储所有的数据
all_data = []
cnt = 0
@@ -57,7 +52,6 @@ def tar_processing(tar_path, output_dir, logger):
with open(os.path.join(filepath, file), 'r') as f:
df = json.load(f)
cnt = cnt + 1
- # 将DataFrame转换为.json格式,并添加到all_data列表中
all_data.extend([df])
dir_name = os.path.basename(filepath)
# write all data to a json file
@@ -73,19 +67,15 @@ def tar_processing(tar_path, output_dir, logger):
def untar(filepath, logger):
- # 如果文件是tar文件,就解压它
if tarfile.is_tarfile(filepath):
- # 创建一个新的文件夹,和tar文件同名,但去掉后缀
new_folder = os.path.splitext(filepath)[0]
tar_name = os.path.basename(filepath)
with tarfile.open(filepath) as tar:
- # 获取tar文件中的所有成员
members = tar.getmembers()
if not os.path.exists(new_folder):
os.mkdir(new_folder)
else:
f = os.listdir(new_folder)
- # 打开tar文件,并解压到新的文件夹中
if len(members) == len(f):
logger.info(f'{tar_name} already decompressed')
return new_folder
@@ -107,32 +97,25 @@ def cp_rm(filepath, output_dir):
os.system('mv -f {} {}'.format(filepath, target_dir))
-parser = argparse.ArgumentParser()
-# parser.add_argument('-d', '--download_json_dir', type=str, default=None)
-parser.add_argument('image_dir', type=str) # grit raw directory
-parser.add_argument('output_dir', type=str) # processed grit output dir
-parser.add_argument('--log_name', type=str, default='grit_processing.log')
-
-args = parser.parse_args()
-
-
def main(args):
logger = create_logger(args.log_name)
- # if args.download_json_dir != None:
- # count_download_image(args.download_json_dir, logger)
- if args.image_dir is not None:
- all_file_name = [
- os.path.join(args.image_dir, file)
- for file in os.listdir(args.image_dir) if file.endswith('.tar')
- ]
- all_file_name.sort()
- func = partial(
- tar_processing, output_dir=args.output_dir, logger=logger)
- with Pool(processes=10) as pool:
- result = pool.imap(func=func, iterable=all_file_name)
- for r in result:
- print(result)
+ all_file_name = [
+ os.path.join(args.image_dir, file)
+ for file in os.listdir(args.image_dir) if file.endswith('.tar')
+ ]
+ all_file_name.sort()
+ func = partial(tar_processing, output_dir=args.output_dir, logger=logger)
+ with Pool(processes=args.num_process) as pool:
+ result = pool.imap(func=func, iterable=all_file_name) # noqa
+ # print(result)
if __name__ == '__main__':
+ parser = argparse.ArgumentParser()
+ parser.add_argument('image_dir', type=str) # grit raw directory
+ parser.add_argument('output_dir', type=str)
+ parser.add_argument('--num-process', default=10)
+ parser.add_argument('--log-name', type=str, default='grit_processing.log')
+ args = parser.parse_args()
+
main(args)